
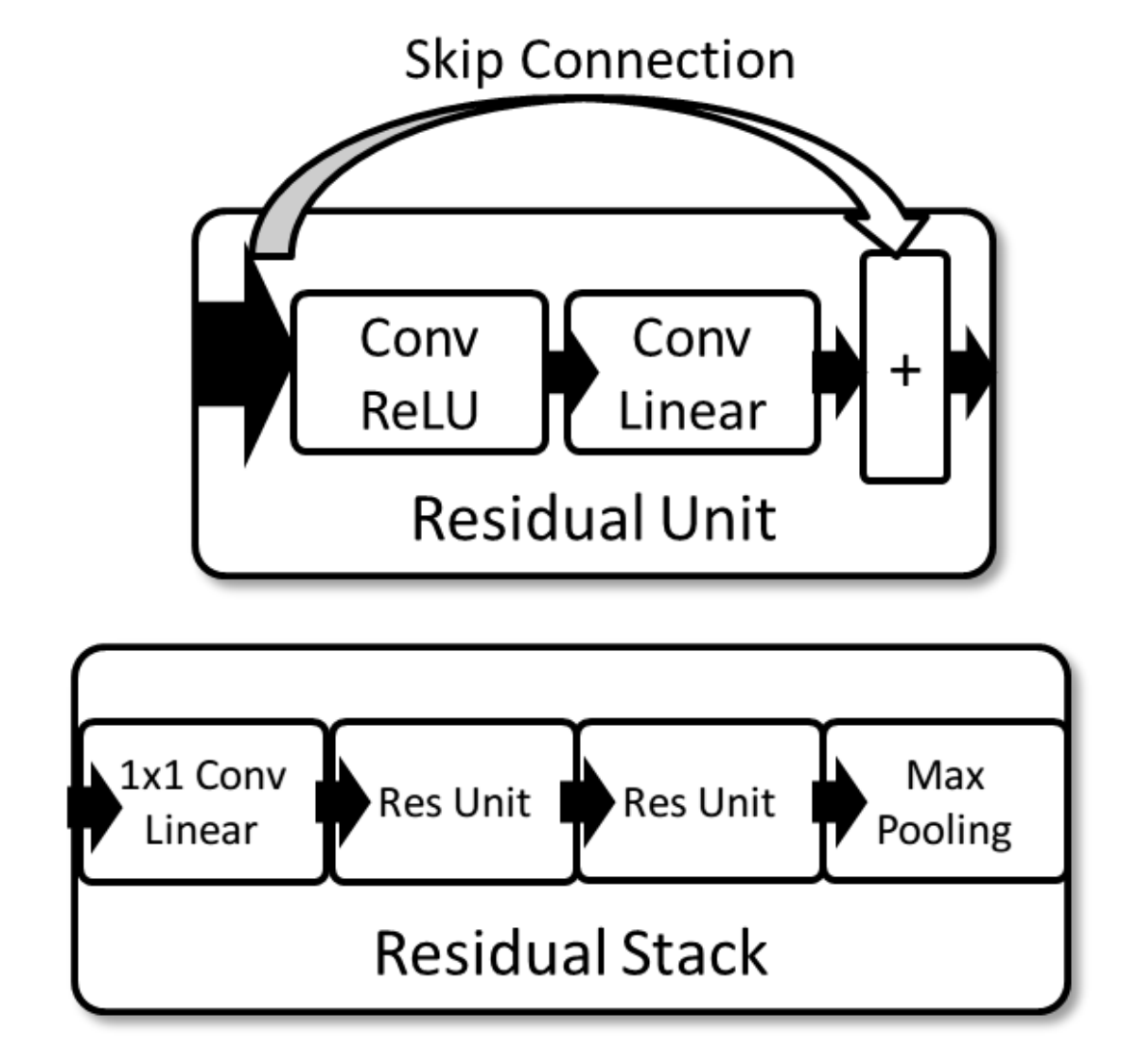
Theoretical analysis shows that the proposed model is able to produce a richer basis function containing both the desired odd- and even-order terms, resulting in improved modeling capability and distortion mitigation.

The input signal of the proposed ARVTDNN consists of Cartesian in-phase and quadrature phase (I/Q) components, as well as envelope-dependent terms. Used as a digital-predistortion algorithm, the best RBFNN with memory suppressed the lower (upper) adjacent channel power 7 dB (4 dB) compared to a memoryless nonlinear predistorter and 11 dB (13 dB) compared to the case of no predistortion for the same output power for a 4-MHz-wide signal.Ī digital predistorter, modeled by an augmented real-valued time-delay neural network (ARVTDNN), has been proposed and found suitable to mitigate the nonlinear distortions of the power amplifier (PA) along with modulator imperfections for a wideband direct-conversion transmitter. For the 20-MHz signal, the models have similar performance in- and out-of-band. For the 4-MHz signal, the RBFNN has better in-band performance, whereas the PH is better out-of-band, when memory is used. The two model types have similar performance when no memory is used. The RBFNN is compared to a parallel Hammerstein (PH) model. Noise-like signals with bandwidths of 4 and 20 MHz were used. Sampled input and output signals were used for identification and validation. The model requires less training than a model using IQ data. In the model, the signal's envelope is used. The presented approach is evaluated on a real LDMOS (laterally diffused metal oxide semiconductor) push-pull power amplifier with 16 MHz bandwidth OFDM (orthogonal frequency-division multIPlex) signal at a carrier frequency of 200 MHz.Ī radial-basis function neural network (RBFNN) has been used for modeling the dynamic nonlinear behavior of an RF power amplifier for third generation. Conversely, the PAPR of the predistorted signals at the output of the TADNN remains moderate. Indeed, one drawback of VoLTErra based predistorters is that they can generate predistorted signals with very high PAPR that cannot be applied directly at the input of the power amplifier. This comparison is based on three elements: performance in linearization, complexity, increase of the peak to average power ratio (PAPR) by the predistorter.
#Residual neural network series#
We also compare the TADNN predistorter with predistorters derived from VoLTErra series such as memory polynomial or dynamic deviation reduction models. We show that the introduction of advance taps improves the digital predistortion (DPD) performance. We name this architecture TADNN (tapped advance and delay line neural net).
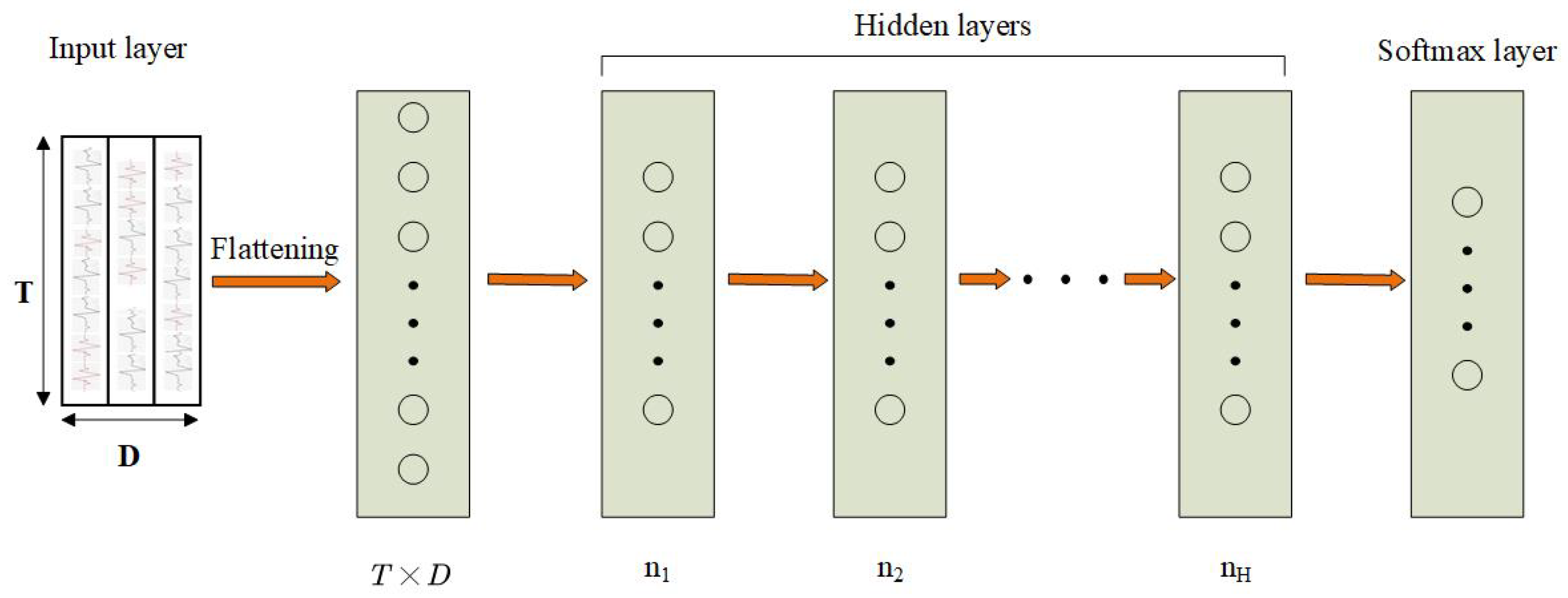
It is based on a feedforward tapped delay line neural network for complex signals with one hidden layer but it includes both delayed and advanced samples at its input. This paper is focused on digital predistortion using neural networks (NNETs) for linearization of power amplifiers.
